Daily Shaarli
March 5, 2024
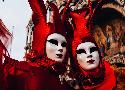
Losing the imitation game
AI cannot develop software for you, but that's not going to stop people from trying to make it happen anyway. And that is going to turn all of the easy software development problems into hard problems.
If you've been anywhere near major news or social media in the last few months, you've probably heard repeatedly about so-called AI, ChatGPT, and large language models (LLMs). The hype surrounding these topics has been intense. And the rhetoric has been manipulative, to say the least. Proponents have claimed that their models are or soon will be generally intelligent, in the way we mean humans are intelligent. They're not. They've claimed that their AI will eliminate whole categories of jobs. And they've claimed that developing these systems further and faster is both necessary and urgent, justified by science fiction dressed up as arguments for some sort of "safety" that I find to be incoherent.
The outer layer of hype surrounding AI—and LLM chatbots in particular—is that they will become indispensable tools of daily work, and entirely replace people in numerous categories of jobs. These claims have included the fields of medicine, law, and education, among others. I think it's nonsense. They imagine self-teaching classrooms and self-diagnosing fitness gadgets. These things will probably not even work as well as self-driving cars, which is to say: only well enough to be dangerous. Of course, that's not stopping people from pushing these fantasies, anyway. But these fields are not my area of expertise. My expertise is in software engineering. We should know better, but software developers are falling victim to the same kind of AI fantasies.
A computer can never be held accountable. Therefore, a computer must never make a management decision.
While the capabilities are fantasy, the dangers are real. These tools have denied people jobs, housing, and welfare. All erroneously. They have denied people bail and parole, in such a racist way it would be comical if it wasn't real. And the actual function of AI in all of these situations is to obscure liability for the harm these decisions cause.
So-Called AI
Artificial Intelligence is an unhelpful term. It serves as a vehicle for people's invalid assumptions. It hand-waves an enormous amount of complexity regarding what "intelligence" even is or means. And it encourages people confuse concepts like cognition, agency, autonomy, sentience, consciousness, and a host of related ideas. However, AI is the vernacular term for this whole concept, so it's the one I'll use. I'll let other people push that boulder, I'm here to push a different one.
Those concepts are not simple ideas, either. Describing them gets into hard questions of psychology, neurology, anthropology, and philosophy. At least. Given that these are domains that the tech field has routinely dismissed as unimportant for decades, maybe it shouldn't be surprising that techies as a group are now completely unprepared to take a critical view of claims about AI.
The Turing Test
Certainly part of how we got here is the Turing test. That is, the pop science reduction of Alan Turing's imitation game. The actual proposal is more substantial. And taking it seriously produces some interesting reading. But the common notion is something like a computer is intelligent if it can reliably pass as human in conversation. I hope seeing it spelled out like that makes it clear how dramatically that overreaches. Still, it's the framework that people have, and it informs our situation. I think the bit that is particularly informative is the focus on natural, conversational language. And also, the deception inherent in the imitation game scenario, but I'll come back to that.
Our understanding of intelligence is a moving target. We only have one meaningful fixed point to work from. We assert that humans are intelligent. Whether anything else is, is not certain. What intelligence itself is, is not certain. Not too long ago, a lot of theory rested on our ability to create and use tools. But then that ability turned out to be not as rare as we thought, and the consensus about the boundaries of intelligence shifted. Lately, it has fallen to our use of abstract language. That brings us back to AI chatbots. We suddenly find ourselves confronted with machines that seem to have a command of the English language that rivals our own. This is unfamiliar territory, and at some level it's reasonable that people will reach for explanations and come up with pop science notions like the Turing test.
Language: any system of formalized symbols, signs, sounds, gestures, or the like used or conceived as a means of communicating thought, emotion, etc.
Language Models
ChatGPT and the like are powered by large language models. Linguistics is certainly an interesting field, and we can learn a lot about ourselves and each other by studying it. But language itself is probably less than you think it is. Language is not comprehension, for example. It's not feeling, or intent, or awareness. It's just a system for communication. Our common lived experiences give us lots of examples that anything which can respond to and produce common language in a sensible-enough way must be intelligent. But that's because only other people have ever been able to do that before. It's actually an incredible leap to assume, based on nothing else, that a machine which does the same thing is also intelligent. It's much more reasonable to question whether the link we assume exists between language and intelligence actually exists. Certainly, we should wonder if the two are as tightly coupled as we thought.
That coupling seems even more improbable when you consider what a language model does, and—more importantly—doesn't consist of. A language model is a statistical model of probability relationships between linguistic tokens. It's not quite this simple, but those tokens can be thought of as words. They might also be multi-word constructs, like names or idioms. You might find "raining cats and dogs" in a large language model, for instance. But you also might not. The model might reproduce that idiom based on probability factors instead. The relationships between these tokens span a large number of parameters. In fact, that's much of what's being referenced when we call a model large. Those parameters represent grammar rules, stylistic patterns, and literally millions of other things.
What those parameters don't represent is anything like knowledge or understanding. That's just not what LLMs do. The model doesn't know what those tokens mean. I want to say it only knows how they're used, but even that is over stating the case, because it doesn't know things. It models how those tokens are used. When the model works on a token like "Jennifer", there are parameters and classifications that capture what we would recognize as things like the fact that it's a name, it has a degree of formality, it's feminine coded, it's common, and so on. But the model doesn't know, or understand, or comprehend anything about that data any more than a spreadsheet containing the same information would understand it.
Mental Models
So, a language model can reproduce patterns of language. And there's no particular reason it would need to be constrained to natural, conversational language, either. Anything that's included in the set of training data is fair game. And it turns out that there's been a lot of digital ink spent on writing software and talking about writing software. Which means those linguistic patterns and relationships can be captured and modeled just like any other. And sure, there are some programming tasks where just a probabilistic assembly of linguistic tokens will produce a result you want. If you prompt ChatGPT to write a python function that fetches a file from S3 and records something about it in DynamoDB, I would bet that it just does, and that the result basically works. But then, if you prompt ChatGPT to write an authorization rule for a new role in your application's proprietary RBAC system, I bet that it again just does, and that the result is useless, or worse.
Programming as Theory Building
Non-trivial software changes over time. The requirements evolve, flaws need to be corrected, the world itself changes and violates assumptions we made in the past, or it just takes longer than one working session to finish. And all the while, that software is running in the real world. All of the design choices taken and not taken throughout development; all of the tradeoffs; all of the assumptions; all of the expected and unexpected situations the software encounters form a hugely complex system that includes both the software itself and the people building it. And that system is continuously changing.
The fundamental task of software development is not writing out the syntax that will execute a program. The task is to build a mental model of that complex system, make sense of it, and manage it over time.
To circle back to AI like ChatGPT, recall what it actually does and doesn't do. It doesn't know things. It doesn't learn, or understand, or reason about things. What it does is probabilistically generate text in response to a prompt. That can work well enough if the context you need to describe the goal is so simple that you can write it down and include it with the prompt. But that's a very small class of essentially trivial problems. What's worse is there's no clear boundary between software development problems that are trivial enough for an LLM to be helpful vs being unhelpful. The LLM doesn't know the difference, either. In fact, the LLM doesn't know the difference between being tasked to write javascript or a haiku, beyond the different parameters each prompt would activate. And it will readily do a bad job of responding to either prompt, with no notion that there even is such a thing as a good or bad response.
Software development is complex, for any non-trivial project. But complexity is hard. Overwhelmingly, when we in the software field talk about developing software, we've dealt with that complexity by ignoring it. We write code samples that fit in a tweet. We reduce interviews to trivia challenges about algorithmic minutia. When we're feeling really ambitious, we break out the todo app. These are contrivances that we make to collapse technical discussions into an amount of context that we can share in the few minutes we have available. But there seem to be a lot of people who either don't understand that or choose to ignore it. They frame the entire process of software development as being equivalent to writing the toy problems and code samples we use among general audiences.
Automating the Easy Part
The intersection of AI hype with that elision of complexity seems to have produced a kind of AI booster fanboy, and they're making personal brands out of convincing people to use AI to automate programming. This is an incredibly bad idea. The hard part of programming is building and maintaining a useful mental model of a complex system. The easy part is writing code. They're positioning this tool as a universal solution, but it's only capable of doing the easy part. And even then, it's not able to do that part reliably. Human engineers will still have to evaluate and review the code that an AI writes. But they'll now have to do it without the benefit of having anyone who understands it. No one can explain it. No one can explain what they were thinking when they wrote it. No one can explain what they expect it to do. Every choice made in writing software is a choice not to do things in a different way. And there will be no one who can explain why they made this choice, and not those others. In part because it wasn't even a decision that was made. It was a probability that was realized.
[A programmer's] education has to emphasize the exercise of theory building, side by side with the acquisition of knowledge of data processing and notations.
But it's worse than AI being merely inadequate for software development. Developing that mental model requires learning about the system. We do that by exploring it. We have to interact with it. We manipulate and change the system, then observe how it responds. We do that by performing the easy, simple programing tasks. Delegating that learning work to machines is the tech equivalent of eating our seed corn. That holds true beyond the scope of any team, or project, or even company. Building those mental models is itself a skill that has to be learned. We do that by doing it, there's not another way. As people, and as a profession, we need the early career jobs so that we can learn how to do the later career ones. Giving those learning opportunities to computers instead of people is profoundly myopic.
Imitation Game
If this is the first time you're hearing or reading these sentiments, that's not too surprising. The marketing hype surrounding AI in recent months has been intense, pervasive, and deceptive. AI is usually cast as being hyper competent, and superhuman. To hear the capitalists who are developing it, AI is powerful, mysterious, dangerous, and inevitable. In reality, it's almost none of those things. I'll grant that AI can be dangerous, but not for the reasons they claim. AI is complicated and misunderstood, and this is by design. They cloak it in rhetoric that's reminiscent of the development of atomic weapons, and they literally treat the research like an arms race.
I'm sure there are many reasons they do this. But one of the effects it has is to obscure the very mundane, serious, and real harms that their AI models are currently perpetuating. Moderating the output of these models depends on armies of low paid and precariously employed human reviewers, mostly in Kenya. They're subjected to the raw, unfiltered linguistic sewage that is the result of training a language model on uncurated text found on the public internet. If ChatGPT doesn't wantonly repeat the very worst of the things you can find on reddit, 4chan, or kiwi farms, that is because it's being dumped on Kenyan gig workers instead.
That's all to say nothing of the violations of intellectual property and basic consent that was required to train the models in the first place. The scale of the theft and exploitation required to build the data sets these models train with is almost inconceivable. And the energy consumption and e-waste produced by these systems is staggering.
All of this is done to automate the creation of writing or media that is designed to deceive people. It's intended to seem like people, or like work done by people. The deception, from both the creators and the AI models themselves, is pervasive. There may be real, productive uses for these kinds of tools. There may be ways to build and deploy them ethically and sustainably. But that's not the situation with the instances we have. AI, as it's been built today, is a tool to sell out our collective futures in order to enrich already wealthy people. They like to frame it as being akin to nuclear science. But we should really see it as being more like fossil fuels
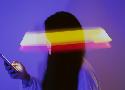
Twitter is becoming a 'ghost town' of bots as AI-generated spam content floods the internet
ABC Science / By technology reporter James Purtill
Parts of the web are now dominated by bots and junk websites designed to go unread by humans.
One morning in January this year, marine scientist Terry Hughes opened X (formerly Twitter) and searched for tweets about the Great Barrier Reef.
"I keep an eye on what's being tweeted about the reef every day," Professor Hughes, a leading coral researcher at James Cook University, said.
What he found that day surprised and confused him; hundreds of bot accounts tweeting the same strange message with slightly different wording.
"Wow, I had no idea that agricultural runoff could have such a devastating impact on the Great Barrier Reef," one account, which otherwise spruiked cryptocurrencies, tweeted.
Another crypto bot wrote: "Wow, it's disheartening to hear about the water pollution challenges Australia faces."
And so on. Hundreds of crypto accounts tweeting about agricultural runoff.
A month later, it happened again. This time, bots were tweeting about "marine debris" threatening the Great Barrier Reef.
What was going on?
When Professor Hughes tweeted what he'd found, some saw a disinformation conspiracy, an attempt to deflect attention from climate change.
The likely answer, however, is more mundane, but also more far-reaching in its implications.
More than a year since Elon Musk bought X with promises to get rid of the bots, the problem is worse than ever, experts say.
And this is one example of a broader problem affecting online spaces.
The internet is filling up with "zombie content" designed to game algorithms and scam humans.
It's becoming a place where bots talk to bots, and search engines crawl a lonely expanse of pages written by artificial intelligence (AI).
Junk websites clog up Google search results. Amazon is awash with nonsense e-books. YouTube has a spam problem.
And this is just a trickle in advance of what's been called the "great AI flood".
Bots liking bots, talking to other bots
But first, let's get back to those reef-tweetin' bots.
Timothy Graham, an expert on X bot networks at the Queensland University of Technology, ran the tweets through a series of bot and AI detectors.
Dr Graham found 100 per cent of the text was AI-generated.
"Overall, it appears to be a crypto bot network using AI to generate its content," he said.
"I suspect that at this stage it's just trying to recruit followers and write content that will age the fake accounts long enough to sell them or use them for another purpose."
That is, the bots probably weren't being directed to tweet about the reef in order to sway public opinion.
Dr Graham suspects these particular bots probably have no human oversight, but are carrying out automated routines intended to out-fox the bot-detection algorithms.
Searching for meaning in their babble was often pointless, he said.
"[Professor Hughes] is trying to interpret it and is quite right to try and make sense of it, but it just chews up attention, and the more engagement they get, the more they are rewarded.
The cacophony of bot-talk degrades the quality of online conversations. They interrupt the humans and waste their time.
"Here's someone who is the foremost research scientist in this space, spending their time trying to work out the modus operandi of these accounts."
In this case, the bots were replying to the tweet of another bot, which, in turn, replied to the tweets of other bots, and so on.
One fake bot account was stacked on top of the other, Dr Graham said.
"It's AI bots all the way down."
How bad is X's bot problem?
In January, a ChatGPT glitch appeared to shine a light on X's bot problem.
For a brief time, some X accounts posted ChatGPT's generic response to requests that it deems outside of its content policy, exposing them as bots that use ChatGPT to generate content.
Users posted videos showing scrolling feeds with numerous accounts stating "I'm sorry, but I cannot provide a response to your request as it goes against OpenAl's content policy."
"Twitter is a ghost town," one user wrote.
But the true scale of X's bot problem is difficult for outsiders to estimate.
Shortly after Mr Musk gained control of X while complaining about bots, X shut down free access to the programming interface that allowed researchers to study this problem.
That left researchers with two options: pay X for access to its data or find another way to peek inside.
Towards the end of last year, Dr Graham and his colleagues at QUT paid X $7,800 from a grant fund to analyse 1 million tweets surrounding the first Republican primary debate.
They found the bot problem was worse than ever, Dr Graham said at the time.
Later studies support this conclusion. Over three days in February, cybersecurity firm CHEQ tracked the proportion of bot traffic from X to its clients' websites.
It found three-quarters of traffic from X was fake, compared to less than 3 per cent of traffic from each of TikTok, Facebook and Instagram.
"Terry Hughes' experience is an example of what's going on on the platform," Dr Graham said.
"One in 10 likes are from a porn bot, anecdotally."
The rise of a bot-making industry
So what's the point of all these bots? What are they doing?
Crypto bots drive up demand for certain coins, porn bots get users to pay for porn websites, disinformation bots peddle fake news, astroturfing bots give the impression of public support, and so on.
Some bots exist purely to increase the follower counts and engagement statistics of paying customers.
A sign of the scale of X's bot problem is the thriving industry in bot-making.
Bot makers from around the world advertise their services on freelancer websites.
Awais Yousaf, a computer scientist in Pakistan, sells "ChatGPT Twitter bots" for $30 to $500, depending on their complexity.
In an interview with the ABC, the 27-year-old from Gujranwala said he could make a "fully fledged" bot that could "like comments on your behalf, make comments, reply to DMs, or even make engaging content according to your specification".
Mr Yousaf's career tracks the rise of the bot-making economy and successive cycles of internet hype.
Having graduated from university five years ago, he joined Pakistan's growing community of IT freelancers from "very poor backgrounds".
Many of the first customers wanted bots to promote cryptocurrencies, which were booming in popularity at the time.
"Then came the NFT thing," he said.
A few years ago he heard about OpenAI's GPT3 language model and took a three-month break to learn about AI.
"Now, almost 90 per cent of the bots I do currently are related to AI in one way or another.
"It can be as simple as people posting AI posts regarding fitness, regarding motivational ideas, or even cryptocurrency predictions."
In five years he's made 120 Twitter bots.
Asked about Mr Musk's promise to "defeat the spam bots," Mr Yousaf smiled.
"It's hard to remove Twitter bots from Twitter because Twitter is mostly bot."
AI-generated spam sites may overwhelm search engines
X's bot problem may be worse than other major platforms, but it's not alone.
A growing "deluge" of AI content is flooding platforms that were "never designed for a world where machines can talk with people convincingly", Dr Graham said.
"It's like you're running a farm and had never heard of a wolf before and then suddenly you have new predators on the scene.
"The platforms have no infrastructure in place. The gates are open."
The past few months have seen several examples of this.
Companies are using AI to rewrite other media outlet's stories, including the ABC's, to then publish them on the company's competing news websites.
A company called Byword claims it stole 3.6 million in "total traffic" from a competitor by copying their site and rewriting 1,800 articles using AI.
"Obituary pirates" are using AI to create YouTube videos of people summarising the obituaries of strangers, sometimes fabricating details about their deaths, in order to capture search traffic.
Authors are reporting what appear to be AI-generated imitations and summaries of their books on Amazon.
Google's search results are getting worse due to spam sites, according to a recent pre-print study by German researchers.
The researchers studies search results for thousands of product-review terms across Google, Bing and DuckDuckGo over the course of a year.
They found that higher-ranked pages tended to have lower text quality but were better designed to game the search ranking algorithm.
"Search engines seem to lose the cat-and-mouse game that is SEO spam," they wrote in the study.
Co-author Matti Wiegman from Bauhaus University, Weimar said this rankings war was likely to get much worse with the advent of AI-generated spam.
"What was previously low-quality content is now very difficult to distinguish from high-quality content," he said.
"As a result, it might become difficult to distinguish between authentic and trustworthy content that is useful and content that is not."
He added that the long-term effects of AI-generated content on search engines was difficult to judge.
AI-generated content could make search more useful, he said.
"One possible direction is that generated content will become better than the low-quality human-made content that dominates some genres in web search, in which case the search utility will increase."
Or the opposite will happen. AI-generated content will overwhelm "vulnerable spaces" such as search engines and "broadcasting-style" social media platforms like X.
In their place, people may turn to "walled gardens" and specialised forums with smaller numbers of human-only members.
Platforms prepare for coming flood
In response to this emerging problem, platforms are trialling different strategies.
Meta recently announced it was building tools to detect and label AI-generated images posted on its Facebook, Instagram and Threads services.
Amazon has limited authors to uploading a maximum of three books to its store each day, although authors say that hasn't solved the problem.
X is trialling a "Not a Bot" program in some countries where it charges new users $1 per year for basic features.
This program operates alongside X's verification system, where users pay $8 per month to have their identity checked and receive a blue tick.
But it appears the bot-makers have found a way around this.
All the reef-tweeting crypto bots Professor Hughes found were verified accounts.
"It's clutter on the platform that's not necessary. You'd wish they'd clean it up," the coral scientist said.
"It wastes everyone's time."